Statistical Learning & Computational Finance Lab
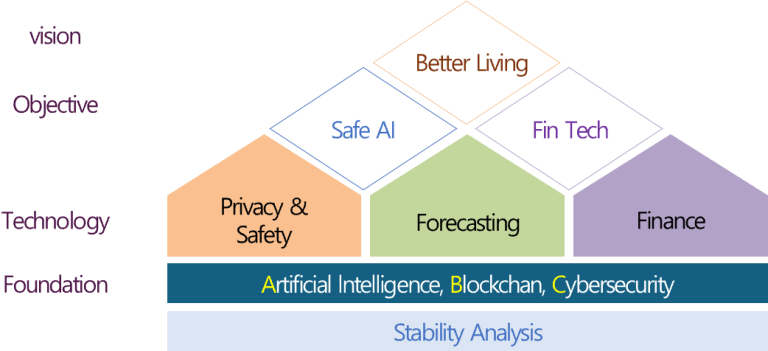
“The Statistical Learning and Computational Finance Lab focuses on ensuring the safety of artificial intelligence systems by
studying ethical fairness, privacy and security, and algorithmic stability and interpretability.
Additionally, based on key fintech domains such as blockchain and cybersecurity,
the lab seeks innovative solutions that challenge traditional financial methods.”
or Projects
Graduate: Applied Multivariate Statistics, Advanced Topics in Statistical Learning, Special Topics in Probability Models
The research focus of this lab is on developing and analyzing efficient algorithms for learning methods, optimization, and inference problems. The research areas can be broadly categorized into three domains: ‘Statistical Learning and Kernel Machines,’ ‘Computational Finance,’ and ‘Global Optimization and Dynamics.’
Statistical Learning and Kernel Machines
Statistical learning involves analyzing and studying machine learning algorithms through the approaches of statistics. This field develops algorithms that enable computers to extract and acquire useful information from large datasets by utilizing various statistical models, kernel techniques, and optimization methods.
Computational Finance
Computational finance is a field that models problems related to financial markets and traded financial products mathematically and solves them using efficient computational methods. To achieve this, various state-of-the-art scientific computation techniques, as well as mathematical and statistical modeling methods, are researched and developed.
Global Optimization and Dynamics
This area involves studying the stability analysis of nonlinear systems and efficiently identifying multiple equilibrium points using dynamic systems theory and advanced topological methodologies. Based on these studies, new global optimization algorithms are developed.